0. Introduction
I sometimes get asked if there are many differences between the languages spoken in North and South Korea, to which I usually answer “not that much”. Since the Sunshine policy era, North-South linguistic differences have given birth to something akin to niche market for dramatic news report, phone apps, books, TV segments and dictionaries. Starting in 2004, scholars in the South have worked on a unified dictionary of the Korean language that would restore the language to its pre-division unity. But all of these initiatives and reports have to be taken with a grain of salt.
The unified dictionary initiative, or “Great Dictionary of our brethren’s language” (kyŏre mal k’ŭn sajŏn), is a case in point. Its very name reveals the underlying linguistic assumptions of the people who claim the division of the Korean language. Indeed, whoever coined that title tried very hard to avoid Sino-Korean words and to replace them with “purely” Korean terms, hence the kyŏre (겨레, literally ‘brethren’, ‘bloodline’) instead of minjok (민족, 民族, nation) and the awkward use of k’ŭn (큰, big) when the Chinese character tae (대, 大, big) is much more common. The contemporary Korean lexicon can be roughly divided into 3 categories, Sino-Korean words which derive from Chinese and can be written with Chinese characters, “pure” Korean words (“순 우리말”, sun uri mal or 고유어 koyuŏ) and loanwords from other languages such as English, Japanese or Russian (외래어, oeraeŏ).
Purist, prescriptive linguists in both North and South have long favored the replacement of Sino-Korean or English loanwords with “Pure” Korean terms. Northerners are usually better than the South at making up “pure” Korean neologisms, favoring, for instance, the word son chŏnhwa (손전화, literally “hand phone”) to say mobile phone when Southerners would use words like 휴대폰 (hyudaep’on, “portable phone”) or 헨드폰 (haendŭp’on, “hand phone”) , keeping the English “phone” in its Korean pronunciation (p’on). The unified dictionary initiative clearly belongs to this category, lamenting on its website that “[t]he tragedy of separation of two Koreas degraded our tongue and the pollution of language has been eroding our collective spirit and threatening our future as one people.”
The problem with this conception of the Korean language is that it is entirely fictitious. There was never a pure, unified Korean language before the separation of the Koreas. There were merely efforts to “unify” the Korean language into a modern national language by imposing the dialect spoken in Seoul as a standard. And while these efforts have been romanticized as acts of resistance to the Japanese colonizer after 1945, the colonial authorities actually played a very strong role in promoting the standardization of Korean and eradicating dialects which it saw as key to “modernizing” Korea. Colonial-era research on a standard Korean language was the basis for the two Koreas’ post-war linguistic policies, which in turn explains the relative proximity of contemporary standard North Korean (문화어, munhwaŏ) and standard South Korean (표준어, p’yojunŏ).
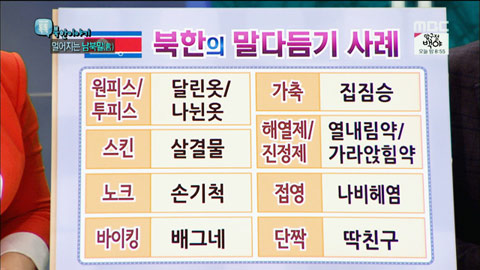
A South Korean speaker, or a foreigner taught South Korean p’yojunŏ would therefore, upon visting Pyongyang, find it fairly easy to communicate. The countryside, however, is another story, as people are less likely to be fluent in the standard dialect than Pyongyang residents. People up in Sinŭiju are hard to understand, but even Pyongyang natives will confess having great trouble understanding the dialect. Not unlike the way Seoul natives are often left bewildered by the Jeju dialect. In fact, the difference between dialects and the official languages is very likely greater than the difference between the official languages themselves.
Claims that the everyday North Korean lexicon is 40% different from the Southern one (and 66% different for technical vocabulary), therefore, can hardly be taken at face value. The vast majority of this gap is likely to come from the minor effects of the different spelling and spacing rules practiced in both countries, such as the omission of initials ㄴ / ㄹ consonants in Southern orthography (the Northern ryŏksa – 력사 becoming yŏksa – 역사). The “purification” practiced by North Korean linguists on foreign loanwords creates lexical differences, but the pure Korean or Sino-Korean neologisms that are created as replacement are quite self transparent. For instance, the English word “knock”, translated in the South as nok’ŭ (노크) becomes son kich’ŏk (손기척) or literally “hand sound-signal” in the North. And just because linguists recommend the use of “pure” Korean words does not mean that people actually use them: in the 1980’s the word ŏrŭm posungi (얼음보숭이, meaning something like ice candy) was introduced as a translation for ‘ice cream’ but people nonetheless kept using the loanwords aisŭk’ŭrim (아이스크림) or esŭk’imo (에스키모, from “eskimo”, a popular moniker for ice cream in Europe and South America).
The North Korean language is actually far less hermetic to foreign loan words than generally thought (Kim Jong-il himself noted that all languages receive the influence of other languages). A word like wŏnjup’il (원주필, 圓珠筆, literally round-ball-pen) sometimes cited as a purification of the loanword polp’aen (볼팬, from the English “ball-pen” through the Japanese bōrupen ボールペン), is actually of Chinese origin. And in technical domains, loanwords have always been common, although their spelling and origins can variate from what people in the South use. The following examples, for computer linguo are quite telling:
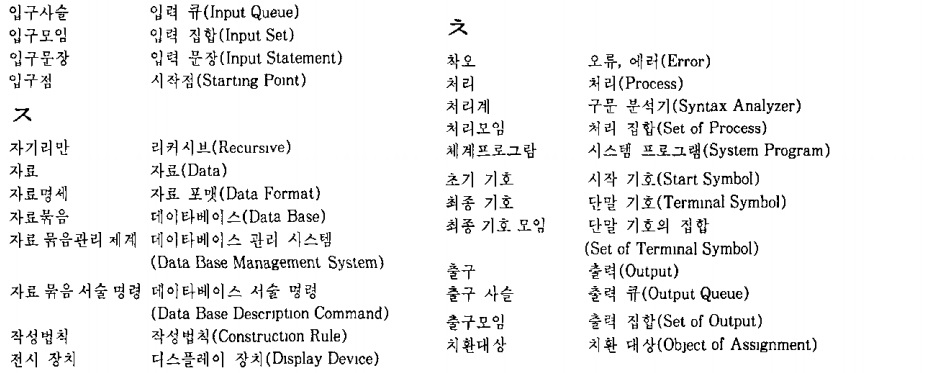
For most technical terms both North and South use a Korean translation rather than a simple Hangul/Chosongul transliteration of the English word. However, while the translations mean the same thing they often use different words. For example, “Set of Terminal Symbols” is ch’oejong kiho moim (최종 기호 모임, “final symbols group”) in the North and tanmal kiho ŭi chiphap (단말 기호의 집합, “gathering of conclusion symbols”) in the South. While technically different, both terms are effectively synonymous and their meaning is self-evident. For several terms, however, the Northern word opts for a Koreanized version when the Southern word is a mere transliteration of the English. This is the case for example of database, which is teit’abeisŭ (데이타베이스) in the South but charyo mugŭm (자료 묶음, “data bundle”) in the North. While in this case a Southern speaker would easily grasp the meaning of the Northern word, it would be harder for a Northerner who does not know English to understand the meaning of the South Korean word. Indeed, the higher prevalence of English loanwords in the South is one of the main linguistic hurdles migrants from the North face after resettling in the South. Finally, we have the case in which both North and South choose to transliterate a foreign word. There are minor spelling discrepancies due to the different ways an English word can be transliterated in Korean, but the words remain easily identifiable (algorithm becomes algoridŭm (알고리듬) in the North but algorijŭm (알고리즘) in the South).
The standard North Korean language and the standard South Korean language are therefore not that different from each other in the sense that they are easily mutually intelligible and that, save some minor spelling differences, they have a very large common lexicon. However, this does mean that the languages are essentially the same. In particular, from a Natural Language Processing point of view, there are a number of very significant differences between the two that need to be adressed.
The most important one might be the different word distributions between the two languages. For instance, there are many different ways to say “girl” in Korean. North Koreans would typically use the word ch’ŏnyŏ (처녀). The word is part the South Korean lexicon too and its meaning would hold no ambiguity for a South Korean speaker. But that same South Korean speaker is more likely to use the term yŏja (여자) when referring to a girl. As a result, the frequency of each term in South and Northern text corpora will be radically different. The differences in spelling and spacing, while seemingly trivial, add to the difficulty of transferring an NLP model from one language to the other. Finally, the main difference is a cultural one: plenty of North Korean terms, especially political ones such as Juche or Songun, are familiar to South Korean speakers because they are regularly discussed in the press. But the way the terms are talked about in the South and the North differs radically. Because context, i.e. the words that cooccur with a term in the same sentence or document, are an essential way to gather semantic information in NLP, this difference likewise poses a challenge.
2. Word Embeddings
Word embeddings have become a very common tool for NLP tasks over the past few years. The technique allows to map words from a vocabulary to vectors of real numbers in an unsupervised way based on the co-occurrence statistics of words in a (preferably) large corpora. There are plenty of great introductions to the topic around and the seminal papers which started the hype so there is no need to go into further detail here. Once vector representations of a vocabulary have been produced from a large corpora, the vectors can be shared and used for a number of different tasks. The idea being that a large enough corpora would be able to capture all the semantic variations of each word in relation to the others.
This property makes word embeddings a valuable tool not only for NLP tasks, but also for discourse analysis and the social sciences in general. Because they offer a literal, spatial map of semantic relationships between the words of a vocabulary, they make it easy to test the relatedness or lack thereof between certain concepts and to explore the implicit assumptions behind our every day language. Two recent articles, for instance, explore how embeddings “acquire historic cultural associations” and thus can reveal various forms of biases. They do so by testing the similarity of certain occupations’ vectors to vectors representing a certain race or gender. There is, however, no reason to focus only on bias: the proximity and distance between various terms can be used to provide a formal overview of the underlying cultural or ideological structure of a work, in the way semioticians like Greimas tried to formalize ideological discourses:
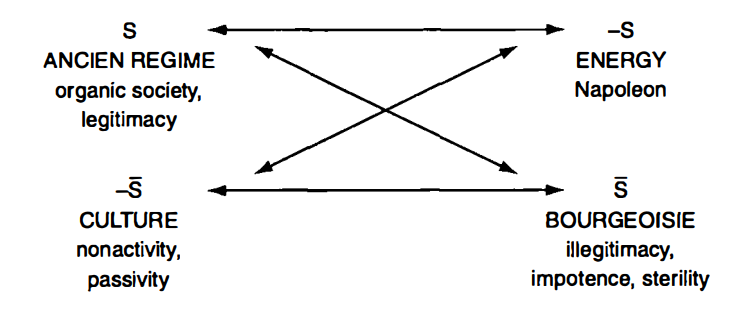
Rather than trying to compile a large comprehensive corpus, one can also use a more restricted, but still sizeable, selection of texts on purpose in order to obtain a specific semantic representations of a vocabulary. For instance the HistWords project at Stanford uses historically distinct corpora to track the semantic change of certain words in different languages :
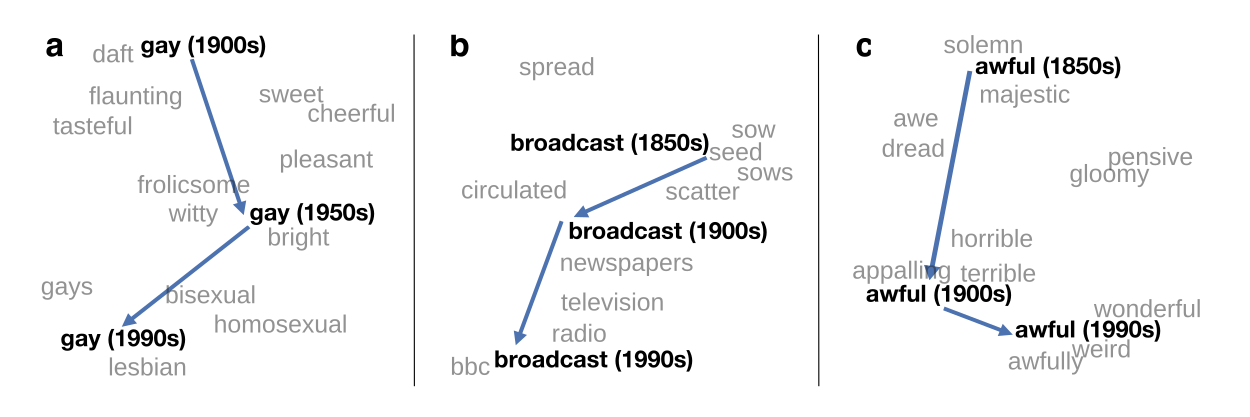
Source: HistWords
Back to Korea: after this brief overview of word embeddings, it should be quite clear that embeddings trained on South Korean data would perform poorly when applied to North Korean texts. The spelling variants would create a lot of out of vocabulary words unless specifically addressed, and the relationships between words would not transfer well due to the cultural differences highlighted above. For this reason, I recently had to train my own word embeddings to use for North Korea-specific NLP tasks. The training corpus was fairly small: 1.1 Gb from 160,000 different documents (newspapers, magazines, novels, political pamphlets and the complete works of Kim Il-Sung) totaling 200 million words. Sentences were split and tokenized using the open-source library Komoran. Since Komoran was trained on South Korean data, it showed some problem splitting up long North Korean compound words. It was therefore not ideal but it nonetheless performed much better and faster than other available libraries. I used gensim to train the model with a vector size of 200 and a window size of 7 on both sides. It is available here.
After playing around with the model, I decided to do some comparisons with a model trained on Google News for the South Korean language (available here), to see what kind of linguistic or cultural differences were captured by the embeddings.
3. Some simple comparisons
Word embeddings make it very easy to analyze semantic proximity and distance between different words using simple arithmetic. We can, for instance, replicate the experiment of Bolukbasi et al. on gender bias and professional occupations. To do so we would have to create separate lists of occupations for North and South as the professional nomenclature between the two countries is fairly different due to economic and cultural reasons. This is easily done by extracting list of n-neighboring vectors for common occupations such as employee, worker, homemaker… from our set of pre-trained vectors for both North and South Korean.
The next step is to create a gender vector by substracting the vectors representing two strongly gendered words. Usually ‘he’ and ‘she’ are used in English, but this is not an option for Korean as the third-person pronoun kŭ (그) is largely gender neutral and doubles as a demonstrative. Meanwhile, the feminine pronoun kŭnyŏ is a modern invention, created to render the use of the feminine third-person pronoun in Western and Japanese novels. The pronoun is getting more popular in South Korea because it is widely used to mirror the use of the English ‘she’ in popular music lyrics or translated movie titles, but it is still hardly ever used in everyday conversation and much less common in the North. To circumvent this, we’ll use other gendered pairs such as mother/father, girl/boy and sum up the difference between their vectors to get an abstract gender vector to which to compare other words’ vector using cosine similarity:
couples_nk = [('어머니', '아버지'), ('할머니', '할아버지'), ('녀자', '남자'), ('딸', '아들'), ('언니', '오빠') ] v_gender_nk = np.sum([model.word_vec(female, use_norm = True) - model.word_vec(male, use_norm = True) for female, male in couples_nk], axis = 0) v_gender_nk = v_gender_nk / np.linalg.norm(v_gender_nk) top_male_jobs = sorted([(model.wv.word_vec(w, use_norm=True).dot(v_gender_nk), w) for w in nkoc if w in model])[:10] top_female_jobs = sorted([(model.wv.word_vec(w, use_norm=True).dot(v_gender_nk), w) for w in nkoc if w in model], reverse = True)[:10]
Doing the same for South Korea, we get the following table:
Female | Male | |
North Korea |
[(0.30784, '간호원 (nurse)'), (0.17584, '선수 (athlete)'), (0.16073, '가수 (singer)'), (0.15478, '운전수 (driver)'), (0.13671, '농장원 (farm worker)'), (0.12744, '종업원 (employee)'), (0.12278, '관리원 (administrative staff)'), (0.10791, '선전원 (propagandist)'), (0.10159, '편집원 (editorial staff)'), (0.09845, '강사 (lecturer)')] |
[(-0.09646, '지식인 (intellectual)'), (-0.07001, '병사 (soldier)'), (-0.05908, '교사 (teacher)'), (-0.05753, '과학자 (scientist)'), (-0.04991, '어민 (fisherman)'), (-0.04566, '기관사 (qualified driver)'), (-0.04070, '대학교수 (univ. professor)'), (-0.03908, '기술자 (technician)'), (-0.03402, '탄부 (coal miner)'), (-0.03156, '광부 (miner)')] |
South Korea |
[(0.130111, '가수 (singer)'), (0.077691, '약사 (pharmacist)'), (0.067639, '간첩 (spy)'), (0.059522, '간호사 (nurse)'), (0.050392, '모델 (model)'), (0.045821, '사진가 (photographer)'), (0.039797, '교사 (teacher)'), (0.015625, '연예인 (entertainer)'), (0.015419, '회사원 (company employee)'), (0.010106, '가정부 (housewife)')] |
[(-0.198744, '정치가 (politician)'), (-0.174848, '군인 (soldier)'), (-0.152985, '발명가 (inventor)'), (-0.132066, '엔지니어 (engineer)'), (-0.125774, '작곡가 (composer)'), (-0.122548, '사장 (company president)'), (-0.111038, '회계사 (accountant)'), (-0.092763, '공무원 (public servant)'), (-0.091915, '기업가 (entrepreneur)'), (-0.090143, '음악가 (musician)')] |
While many of the results won’t come as a surprise, there are nonetheless some interesting finds. The difference between ‘teacher’ being a male job in the North and ‘female’ in the South reflects the fact that primary and secondary level teaching has become increasingly feminized and is now perceived as a ‘female job’ in the South. In the North, on the other hand, because it requires a higher education, the job is still considered an intellectual profession, a category largely associated with men.
The presence of ‘spy’ on the list of female occupations in South Korea should surprise no one familiar with the South Korean media’s obsession with female North Korean spies: from the famous cases of Wŏn Chŏng-hwa and Kim Hyŏn-hŭi to rumors of North Korean beauties targetting South Korean men on social media.
Driving might not actually be a majority female occupation in the North, but female drivers and “tractor girls” have long been part of the country’s popular imagery. It’s worth noting however that while the generic term for driver, unjŏnsu (운전수), is associated with women, the more specific term kigwansa (기관사) denoting higher technical skills, is associated with men.
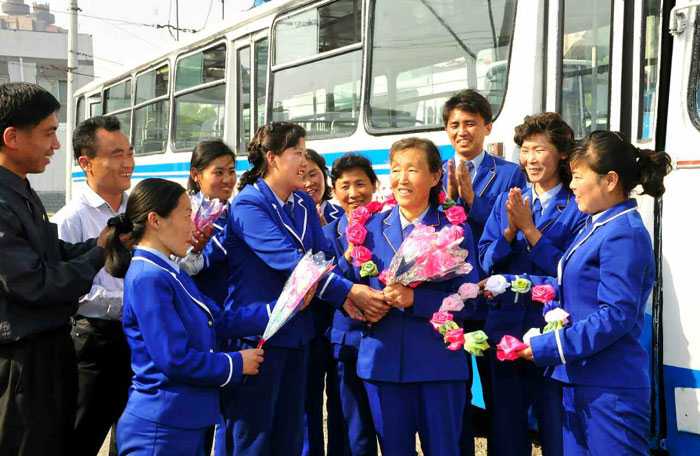
(Source: DPRK Today)
Gender is not the only binary we can explore though. Let’s have a look at what makes North Koreans and South Koreans happy. We define a happiness vector by subtracting the vector for misery (불행) from that of happiness (행복) and list the first 30 closest word vectors from a list of common words for each country:
North Korea | South Korea |
[(0.50366473, '행복'), (0.38692263, '보람'), (0.35191804, '삶'), (0.35147712, '웃음꽃'), (0.35050941, '청춘'), (0.33091822, '강성대국'), (0.32668999, '만복'), (0.32189748, '강성'), (0.30436271, '무릉도원'), (0.30091745, '부강'), (0.29985523, '보금자리'), (0.29675031, '웃음소리'), (0.29325217, '격조'), (0.28211424, '식탁'), (0.28103709, '풍년'), (0.28067306, '축포'), (0.28025869, '요람'), (0.27991235, '강성국가'), (0.27903968, '희열'), (0.27894396, '기쁨'), (0.27587646, '선군조선'), (0.27439246, '휴식'), (0.27419284, '보답'), (0.27290693, '품속'), (0.27058628, '정답'), (0.26619709, '향유'), (0.26605278, '사회주의문화'), (0.26573482, '으뜸'), (0.26431865, '해빛'), (0.26428646, '최상')] |
[(0.47843182, '행복'), (0.28887671, '자유'), (0.28727353, '평등'), (0.26164439, '국민'), (0.25548872, '추구'), (0.25410962, '공공'), (0.25240281, '존엄'), (0.24383035, '설립'), (0.23998213, '동산'), (0.23977825, '소비자'), (0.236155, '인권'), (0.23580489, '청소년'), (0.23320302, '기본'), (0.23280287, '규약'), (0.23257284, '광고'), (0.23155451, '가치'), (0.23109847, '태권도'), (0.22933233, '주거'), (0.22930758, '제고'), (0.22930652, '캠페인'), (0.22856872, '실현'), (0.22298521, '승마'), (0.2213082, '보장'), (0.22064833, '스키장'), (0.21545193, '새'), (0.2153528, '심사'), (0.21486527, '장애인'), (0.21204072, '신설'), (0.21189328, '브랜드'), (0.21160296, '권리')] |
The North Korean list features fairly typical symbols of happiness such as laughter, sunlight or the martial “cannon salute” (축포), a whole group of terms denoting abundance and comfort: good harvest (풍년), dinner table (식탁), nest (보금자리), rest (휴식), and, of course, a number of political slogans : Strong and Prosperous Nation (강성대국, 강성국가), Songun Korea or “socialist culture” (사회주의문화). By contrast, the South Korean list features more abstract political concepts such as equality (평등), community (공공) and human rights (인권), consumerist terms such as brands (브랜드), consumer (소비자), assets (동산) and advertisement (광고) and a number of hobbies: horseriding (승마), skiing (스키장) or Tae Kwon-do (택권도). It’s interesting to see the importance of the political, albeit under very different avatars, in both countries’ definition of happiness.
Let’s have a long at ideas of tradition and modernity :
Traditional | Modern | |
North Korea |
[(0.59895921, '전통'), (0.41555399, '혈통'), (0.3723014, '계승'), (0.36502716, '풍속'), (0.32424557, '유산'), (0.31109792, '문화유산'), (0.31068417, '넋'), (0.31058186, '가문'), (0.31049541, '풍습'), (0.27458504, '조선민족'), (0.27408576, '초석'), (0.27121949, '항일'), (0.26542753, '성산'), (0.26315457, '슬기'), (0.26097465, '자손'), (0.25224674, '고수'), (0.24958429, '실록')] |
[(-0.59895933, '현대'), (-0.36945191, '현대화'), (-0.34926105, '설비'), (-0.32960835, '확장'), (-0.32710972, '신설'), (-0.32017201, '장비'), (-0.30042186, '무인'), (-0.29754138, '최신'), (-0.2965959, '기계'), (-0.29131177, '농기계'), (-0.2776736, '시설물'), (-0.27671432, '생산'), (-0.27605829, '자동화'), (-0.26344311, '공장'), (-0.25346902, '수정주의자 (-0.24700066, '냉동'), (-0.24243492, '기계화')] |
South Korea |
[(0.33539081, '가무'), (0.32670486, '풍습'), (0.3185778, '음식'), (0.30896857, '신앙'), (0.30710441, '습관'), (0.30055016, '참기름'), (0.29570556, '종교적'), (0.29458669, '민속'), (0.29208231, '그릇'), (0.28493643, '떡'), (0.28485328, '정통'), (0.27803272, '전통문화') (0.27406463, '국수'), (0.27146581, '전례'), (0.27018353, '축제'), (0.26900661, '가르침'), (0.26899338, '민속놀이') |
[(-0.55290234, '현대'), (-0.32812071, '대우'), (-0.30197203, '중공업'), (-0.27978188, '코치'), (-0.25041941, '금성'), (-0.24727555, '호랑이'), (-0.22840986, '자동차'), (-0.22042282, '입사'), (-0.21954529, '일화'), (-0.21700546, '사장'), (-0.21508537, '신형'), (-0.21345781, '천안'), (-0.2125137, '증권사'), (-0.20647399, '상무'), (-0.2058209, '아파트'), (-0.2018093, '대기업'), (-0.19441968, '빌딩')] |
North Korea’s conception of tradition emphasizes ancestors and bloodline with words like family/clan (가문), Korean nation (조선민족), bloodline (혈통), descendant (자손), 계승 (succession), 유산 (heritage) but the genealogy works both on a national level and in reference to the Kim’s family. For instance, the word “sacred montain” (성산), for Mount Paektu, refers both to the mythical birthplace of the nation and the alleged center of Kim Il-Sung’s anti-Japanese guerillas. In South Korea, on the other hand, the main theme associated with tradition is food with words such as noodles (국수), rice cake (떡), bowl (그릇), food (음식) and sesame oil (참기름).
The South Korean conception of modernity seems tightly linked to what are often perceived as the pillars of economic growth : big corporations and real estate. For the former, we have words such as the name of former conglomerate Daewoo (대우, Hyundai (현대) is there too but the name itself means ‘modern’ so that is hardly surprising), Goldstar (금성, LG’s former name), security corporation (증권사), big corporation (대기업), business (상무) and heavy industry (중공업). For the latter we have the words building (빌딩) and apartment (아파트). In North Korea too, modernity is an economic phenomenon, but one that is linked to mechanization and automation, with words such as automation (자동화), machinery (기계), mechanization (기계화), agricultural machinery (농기계), automated (무인, literally ‘unmanned’).
4. Let us compare ideologies
Word embeddings also let us quickly visualize the relationships between words. By projecting each vector’s coordinates on a two-dimensional space we can get a scatter plot in which words will be clustered by their semantic proximity. Using such a technique, we can map the way North and South Korea speak about various political ideologies, including their own. We define a list of 13 political ideologies (capitalism, communism, socialism, liberalism, totalitarianism, militarism, individualism, imperialism, colonialism, fascism, anarchism, patriotism and nationalism), list their normalized vector in a matrix on which we perform principal component analysis to reduce the dimensions to 2:
pol_kr = ['자본주의', '공산주의', '사회주의', '자유주의', '전체주의', '군국주의', '개인주의', '제국주의', '식민주의', '파시즘', '무정부주의', '애국주의', '민족주의'] KoreanFont = FontProperties(fname = 'C:\\Windows\\Fonts\\batang.ttc') arr = np.empty((0,200), dtype='f') labels = [] for word in pol_kr: arr = np.append(arr, np.array([model.word_vec(word) / np.linalg.norm(model.word_vec(word))]), axis=0) pca = PCA(n_components=2, random_state=0) Y = pca.fit_transform(arr) x_coords = Y[:, 0] y_coords = Y[:, 1] plt.figure(figsize=(12,6)) plt.scatter(x_coords, y_coords) texts = [] for label, label_en, x, y in zip(pol_kr, pol_en, x_coords, y_coords): texts.append(plt.annotate(label, xy=(x + 0.02, y + 0.01), xytext=(0, 0), textcoords='offset points', ha = 'center', fontproperties = KoreanFont, fontsize = 12)) plt.xlim(x_coords.min()*1.3, x_coords.max()*1.3) plt.ylim(y_coords.min()*1.3, y_coords.max()*1.3) plt.show()
The resulting graphs give us an interesting comparison of the two countries’ political imaginary. In North Korea, socialism, communism and patriotism are clustered together. Nationalism is a bit further apart, and equidistant from totalitarianism. This might be explained by the fact that while nationalism (민족주의) is generally a positive word in North Korean discourse, it is also often used in opposition to communism/socialism and in a negative way when describing the factions of political rivals such as Kim Koo, Cho Man-sik or Syngman Rhee during the colonial and post-liberation period. It would be interesting to further track the polysemy and historical semantic change underwent by the term. Capitalism is unsurprisingly clustered with imperialism, militarism, fascism and colonialism while liberalism (자유주의), closer in meaning to libertarianism in North Korea, gets clustered with anarchism.
In the South on the other hand, liberalism is tightly clustered with individualism and capitalism. Communism, socialism and anarchism are grouped together as all three often tend to be perceived as far-left ideologies. The close association between nationalism, patriotism and fascism is quite unexpected for a country where nationalism is still regarded positively. My guess would be that it is the word “nation” and the adjective “national” that benefit from a positive connotation, while the more academic “nationalism” is used as a critical, negative term:
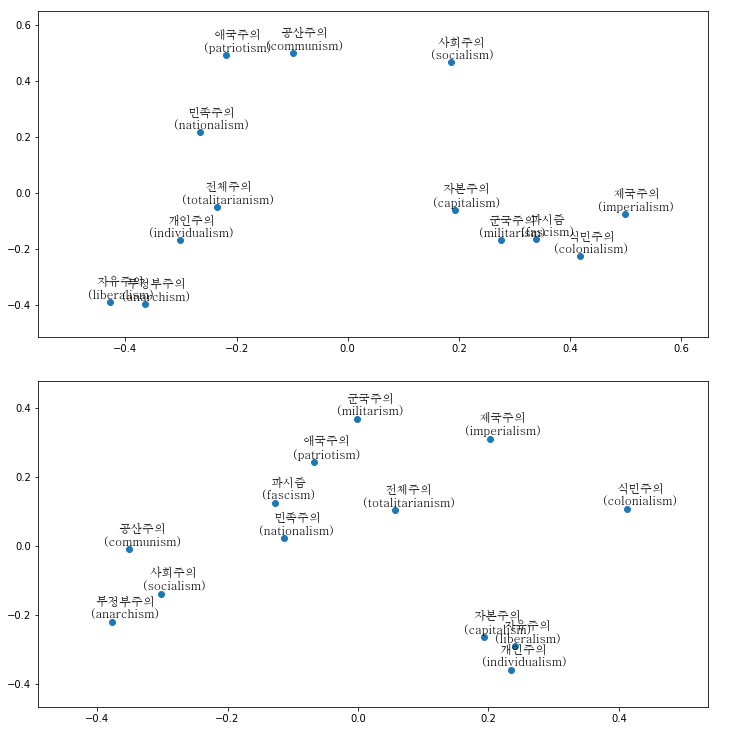
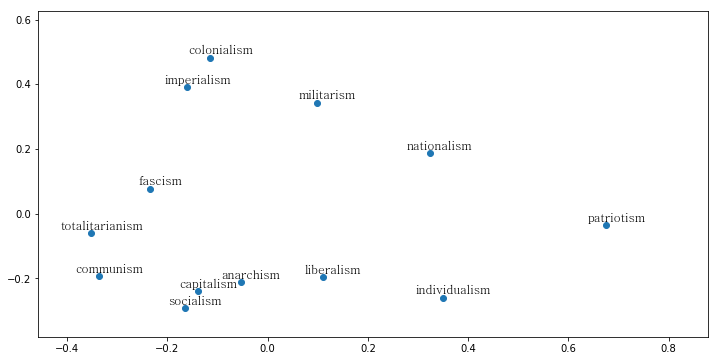
In a 2013 article, Mikolov et al notice that the geometric arrangement of words in vector spaces is relatively similar across languages, something which can be intuitively explained by the fact that “all common languages share concepts that are grounded in the real world (such as that cat is an animal smaller than a dog)”. Based upon this observation, they suggest that it is possible to map word vectors from the source language’s vector space to the target language’s vector space using a simple linear translation.
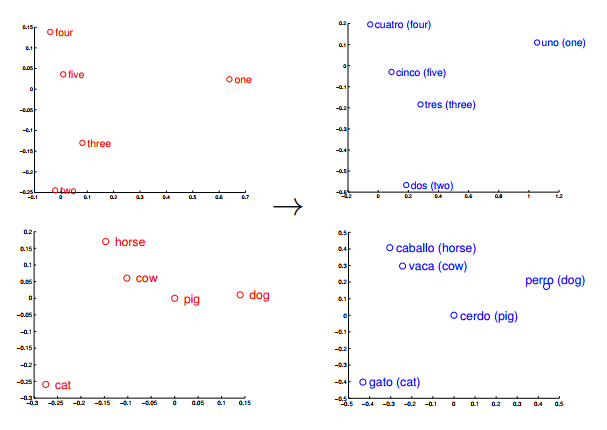
From Mikolov et al., Exploiting Similarities among Languages for Machine Translation (2013).
Given a limited number of pairs of word embeddings {}
where
is the vector representation of word i in the source language and
the vector of its equivalent translation in the target language, the goal is to find a matrix
such that
can approximate
. Once that matrix has been found, any word from the source language can be translated into the target language by taking its vector representation
, multiplying by
, finding the word vector
that is the closest to
in the target vector space and outputting the corresponding target language word.
Mikolov et al.’s idea makes sense since many words do share “objective” semantic characteristic across languages and therefore tend to cluster in a geometrically similar way in the vector space. However, this property is likely to be restricted only to very common words which refer to real-world objects but might not transfer to more abstract concepts. It seems that cross-lingual embeddings models are usually evaluated based on a few thousands of a language’s most common words. I wonder, however, what would happen to the rarer, more ‘subjective’ or politically charged words and if they would indeed be translatable using a simple linear transformation. Definitely something to look into in the future!